Parallels and divergences in spoken word recognition between humans and ASR systems
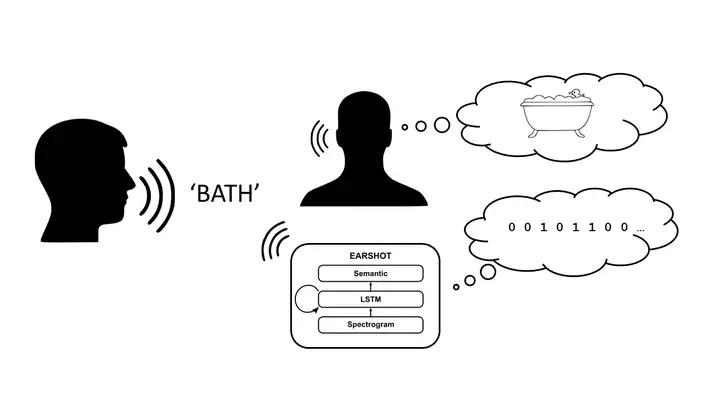
Recent advances in artificial neural networks have enabled the design of automatic speech recognition systems that perform comparably to human listeners in various speech recognition tasks. Careful analysis of the behavioural characteristics of such systems can reveal similarities and critical divergences between machine and human speech recognition. These insights may inspire testable hypotheses using purpose-built artificial neural networks, human psycholinguistic or neuroimaging experiments, and eventually further our understanding of speech perception.
We are using a recently published end-to-end model of human speech recognition, coined EARSHOT Magnuson et al. (2020) and comparing its behavioural characteristics to various aspects of human spoken word recognition: changing speech rates, speakers and levels of acoustic degradation.
So far, we found that the network model responds similarly to humans to speech rate changes, but not to speaker changes. The model also replicates aspects human’s adaptation to degradation of the speech signal. We are investigating which architectural features could explain the lack of speaker change effects and plan follow-up simulation and behavioural experiments. We are also planning manifold geometry analyses and dynamical systems analyses to understand the internal computations ot these systems and compare those to the neural computations in the human speech processing hierarchy.
In collaboration with Matt Davis (MRC-CBU Programme Leader)